As I alluded to in my previous post, we have entered the Moneyball era in tennis. Point length, shot speed, momentum, you name it – more is being measured every day. They say “measure what matters” – but let’s remember that just because we measured it doesn’t mean it mattered. There is risk in rushing to conclusions, and I’ll outline a few considerations for making sure we don’t misinterpret the data we’re collecting.
1. Statistics are situational
Let’s take, for example, something I hear quite often: “the most effective forehand is the inside-out forehand." This conclusion usually comes from statistics showing that more forehand winners are hit from the ad court (for a righty) than from the deuce court. The implication is that players should try to hit more inside-out forehands. But before we take that and run with it, let’s consider the situation. When are players most likely to hit forehands inside-out? For them to be able to run around the ball means that it was probably slower, and for them to feel that their forehand would do more damage than their backhand means it was probably shorter and higher. The result? Most inside-out forehands are hit on slower, shorter, higher balls. Compare that to forehands hit from the deuce court. Even if we only look at the attacks, we’re still counting low balls, wide balls, approach shots, etc – in other words, the more difficult forehands that still fall into the “attacking” category. With that in mind, of course the forehands hit from the ad court are going to be more effective than those hit from the deuce court! Is the inside-out forehand a great weapon? Absolutely. Should players look to use it? Absolutely. But should players be looking to use it more? That depends on how much and when they’re using it already. Telling a player to hit more inside-out forehands just to see them run around balls they cannot attack will only weaken their court position and leave them exposed.
One more hypothetical: say my player is winning 75% of net points. I could look at that and conclude that they should come to the net more, but let’s consider the situation. If my player has won six out of eight net points, it could be that those six were points where they got such an easy ball that they had to come to the net, and the resulting volley was a sitter. If they choose to come to the net more often, they could end up coming in on balls that are more difficult and losing more points.
2. Statistics are player-dependent
What works for one player may not work for another. I might gather some data from the top juniors in the world to present to a player – but what if they have different game styles? The on-paper differences between players of similar levels can be astronomical – net points, aces, double faults, winners to unforced errors, shot placement and more can all vary tremendously. That’s why we have to look up from the paper every now and then to see our players with our own eyes – to know what works for them and what doesn’t, and what will limit them in the future and what won’t.
Similarly, what works against one opponent may not work against another. My player plays a match and wins a sizeable portion of points by taking her backhand down the line. Does that mean she should do it more often? It depends. Maybe this is a strength of hers, and she should take advantage of it. But maybe because of the way she hits it, it’s only effective against certain game styles. Or, maybe it was just effective against this particular opponent due to a weakness they had. The data is useful, but I need more of it, combined with a subjective look at the situation, to come to any impactful conclusions.
3. Correlation does not imply causation
This is, in my opinion, one of the biggest mistakes we can make interpreting data. The saying “correlation does not imply causation” is common in the world of statistics. Simply put, it means that just because two measurements seem linked (see, for example, the fact that murders in NYC rise at a similar rate to ice cream sales) does not mean that one caused the other, and if there is a connection, it certainly doesn’t explain which one caused which. Once again, let’s take an example – this time from a podcast I listened to recently: Djokovic wins, on average, 2.7 games out of the first four. This figure was used to show that Djokovic starts his matches extremely well. I’m not going to dispute that, but consider this: Djokovic is the number 1 player in the world. Most of the matches he plays are against players he is objectively better than. It makes sense, therefore, that he would win more games (across the whole match) than he would lose. Therefore, he might be winning 2.7 games in the first four not because he is a particularly good starter, but because he is simply better than most of the players he plays against. Now, if we find out that he wins more games in the first four than in any other stretch of four, or, better yet, find out that he wins more games in the first four than Nadal or Federer, then we have a case – and I’m not opposed to that. But if I go play my nephew, I can promise you I’ll win the first four games – and it’s not because I’m a good starter.
Take a look at the statistics for any match of your choice and look at second serve points won. Roughly 90% of the time, the player who wins more second serve points wins the match. Crazy, right? Is this the golden statistic? Is this the key to winning in tennis? No. Players aren’t winning matches because they are winning more second serve points – they are winning more second serve points because they are winning the match.
Conclusion
Let me be clear: I am not against stats in tennis, and certainly not against coaches and analysts trying to interpret those stats to form meaningful conclusions. I am merely making two suggestions. First, that we need complete and specific statistics that can be compared against a wide array of players, opponents, and situations. And second, that we must exercise caution in interpreting those stats and think outside the box to consider what the numbers are not telling us. Remember: correlation does not imply causation.
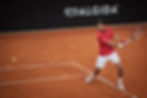
(photo: http://robertofaccenda.it)